COVID-19 in South Korea: A Deep Dive
South Korea was a pandemic poster child—early cases, quick moves, tons of data. I dug into
11 datasets to unpack how COVID-19 hit, spread, and got tamed there. From cases and demographics to weather and
policies, it's a full-on look at what went down and why.
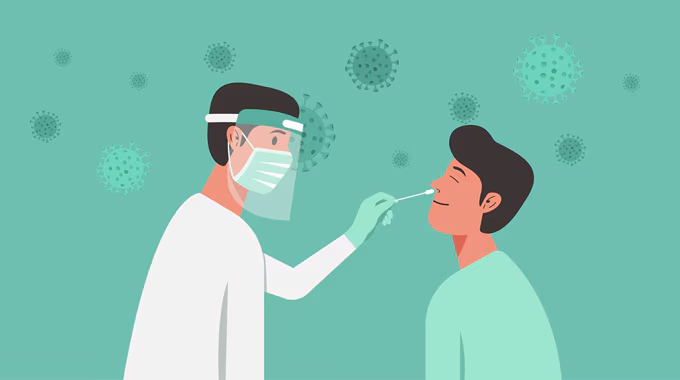
What I Did
- Data Prep: Loaded 11 datasets—cases, patients, timelines, regions, you name it. Cleaned up missing bits and funky formats.
- Exploration: Asked the biggies: How'd it spread? Who got hit hardest? Did policies work? Used maps, charts, and stats to find out.
- Analysis: Tracked trends over time, mapped hotspots, checked demographics, and tested stuff like weather's role.
- Results: Pieced together patterns—like Daegu's wild spike—and gauged what stopped the virus in its tracks.
How It Went
- Data Setup: 11 tables—cases (174 rows), patients (5k+), time series, weather, policies, more. Some gaps (like 4k missing symptom dates), but mostly solid after cleanup.
- Spread: Daegu was ground zero—6,680 cases, Nam-gu alone at 4,511. Group infections (71%) ruled—think churches, clubs—over solo cases. Overseas inflows and close contacts fueled it too.
- Demographics: 20s led confirmed cases (post-imputation, females edged out), but 60s+ took the mortality hit. Men died more despite fewer cases—maybe smoking or heart issues.
- Time: Cases peaked February 2020, releases climbed by March, deaths stayed low-ish. Tests shot up fast—smart move.
- Places: Daegu dwarfed Seoul (1,280 cases). Smaller provinces dodged the worst. Seoul's floating crowd spiked in February—outbreak chaos?
- Weather: Temp vs. cases? Barely a whisper (correlation 0.015)—no big link.
- Policies: Alerts (Level 4 by Feb 23), school shifts, bar bans—tight stuff rolled out fast. Searches for "coronavirus" spiked in March, showing people were dialed in.
The Details
- Data: 174 cases, 5,166 patients, daily stats from Jan 2020. Lat/long mapped, provinces coded, policies dated.
- Stats: Group cases 71% (124/174), Daegu's 6,680 vs. Incheon's 202. Age 20s at 24% confirmed, 80s at 50% deceased (TimeAge). Temp correlation 0.015—meh.
- Trends: Feb peak (daily confirmed ~800), March releases soared, searches hit 100 in March.
What's Next
- Dig deeper into group hotspots—why churches over clubs?
- Cross-check weather with humidity or UV—might tease out more.
- Model policy timing vs. case drops—pinpoint the winners.
Why It Matters
South Korea's story is a playbook: Daegu got slammed (group spread), young folks carried it, older ones bore the brunt. Fast testing and lockdowns crushed it—cases fell while releases rose. Small firms talked mental health more, big ones had resources. It's a blueprint for tackling the next big bug. Maps and charts are on my GitHub—dive in!
What the Data Showed