Podcast Reviews: What's the Buzz?
I dove into a massive dataset of 2 million podcast reviews across 100,000 shows to see
what's clicking with listeners. Stored in a SQLite database with tables for categories, podcasts, reviews, and
runs, it's a goldmine of listener vibes. The goal? Figure out what makes a podcast pop, how ratings shake out,
and what's behind the chatter.
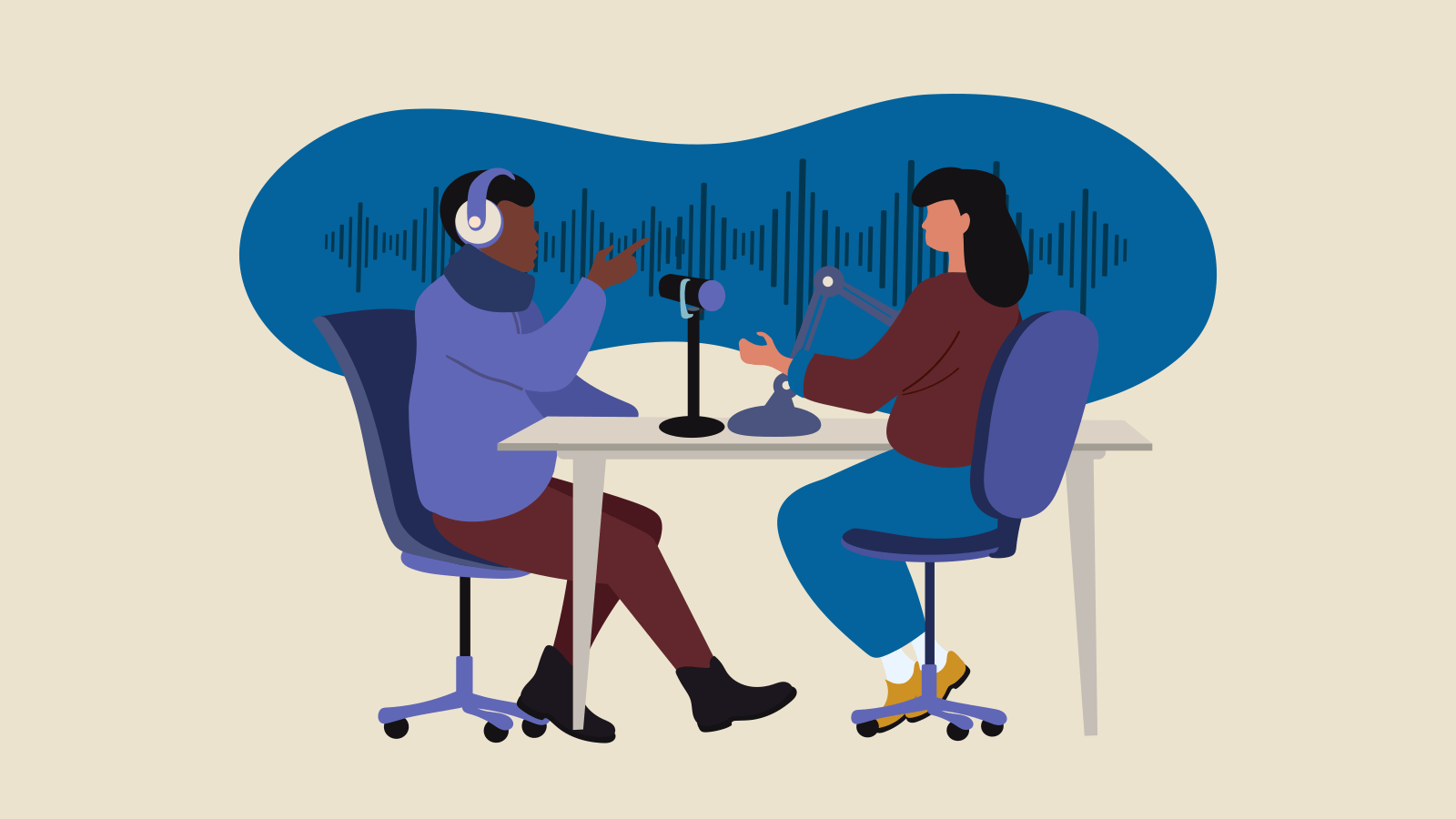
What I Did
- Data Prep: Pulled the data with SQLite and Pandas, scrubbed it clean—dropped duplicates, fixed dates, and added a review length column.
- Exploration: Asked big questions: Which shows get the most reviews? Do long reviews mean high ratings? What's hot by category or over time? Used charts to spot trends.
- Stats Check: Tested hypotheses—like whether review length ties to ratings—with tools like ANOVA.
- Sentiment Peek: Dug into review text to see what words pop up in raves vs. rants.
- Results: Found top podcasts, rating patterns, and even seasonal quirks—all laid out in a Looker dashboard.
How It Went
- Data Setup: Started with 2 million reviews, axed 655 duplicates. Cleaned text (lowercase, no punctuation, no stopwords) and added review lengths. Four tables: categories (212k rows), podcasts (110k), reviews (2M), and runs (16). No gaps—smooth sailing.
- Popularity: "Crime Junkie" crushed it with the most reviews—super popular. But review count vs. average rating? No link (correlation -0.08). Fame doesn't mean five stars.
- Review Length: Tested if longer reviews mean better ratings. ANOVA said yes (p ≈ 0, F = 232)—higher ratings often come with more words, though it's messy with overlap.
- Ratings: 86.7% of reviews are 5 stars—listeners are mostly stoked. Low scores (1-4) are rare, under 6% each.
- Sentiment: Positive reviews gush "love" and "great"; negative ones lean on "get" and "I'm"—not bad words, just griping vibes.
- Categories: "Society-Culture" rules with 16k+ podcasts and tons of 5-star reviews. "TV-Film" and "Sports" mix it up more—ratings all over.
- Time Trends: Reviews spiked in June 2020, ratings peaked in 2018 then dipped. January's review-heavy (164k), December's light (136k). February's tops for ratings (4.66), December's lowest (4.61).
- Authors: Top reviewer (ID D3307ADEFFA285C) dropped 612 reviews—four of the top five stick to 5 stars, one (4.04) mixes it up. They love "Business," "Comedy," and "Education."
The Details
- Data: 2M reviews, 110k podcasts, 212k category tags. Ratings 1-5 (mean 4.63).
- Stats: Review length vs. rating—F = 232, p < 10^-198, big differences across ratings. No review count-rating correlation (-0.08).
- Sentiment: "Love" (426k in positives) vs. "get" (19k in negatives)—clear sentiment split.
- Trends: January reviews 164,907, February avg 4.66—seasonal swings are real but subtle.
What's Next
- Chat with top reviewers—why so many? Maybe a quick survey.
- Go deeper with NLP—pull themes or predict hits from text.
- Model it—could ratings or trends forecast what's next?
Why It's Cool
This cracks open what listeners love: "Crime Junkie" rules, 5-star reviews dominate, and "Society-Culture" shines. Podcasters can see what sticks—longer reviews signal fans, "Business" draws chatterboxes. It's a snapshot of the scene, perfect for creators or platforms to tweak their game. Check the Looker dashboard or GitHub for the full scoop!
What the Data Showed